Solar energy is a key asset in the fight against climate change—but its reliability is challenged by the unpredictable nature of sunlight. A new AI-based model developed by Hungarian researchers offers a game-changing solution: it predicts solar irradiance with high accuracy minutes ahead, using minimal computing power. This innovation could greatly enhance the stability of green power grids and the efficiency of solar energy systems worldwide.
Solar panels play a powerful role in combating climate change, yet their effectiveness is limited by the intermittent nature of sunlight. Sudden cloud cover or shifting weather can cause unpredictable drops in solar energy production, making it harder for power grids to stay balanced.
A recent research by the Environmental Physics Department represents a significant advancement, potentially offering an innovative solution. Researchers from the HUN-REN Center for Energy Research and Budapest University of Technology has developed a lightweight, artificial intelligence-based model that can forecast solar irradiance with high precision just minutes ahead. The innovation uses images of the sky taken every minute by a rooftop camera, combined with detailed weather data.
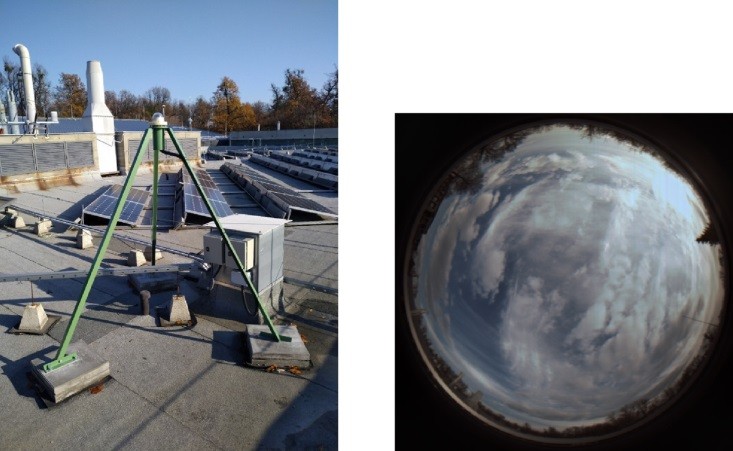
What makes this model stand out is its ability to make quick and accurate predictions while using far fewer computing resources than traditional methods. Instead of analyzing full sky images, it extracts key features—like cloud patterns and sun position—making it 4,000 times less data-heavy. Despite this efficiency, the model achieved a forecast accuracy that outperforms even more complex neural networks in most tests.
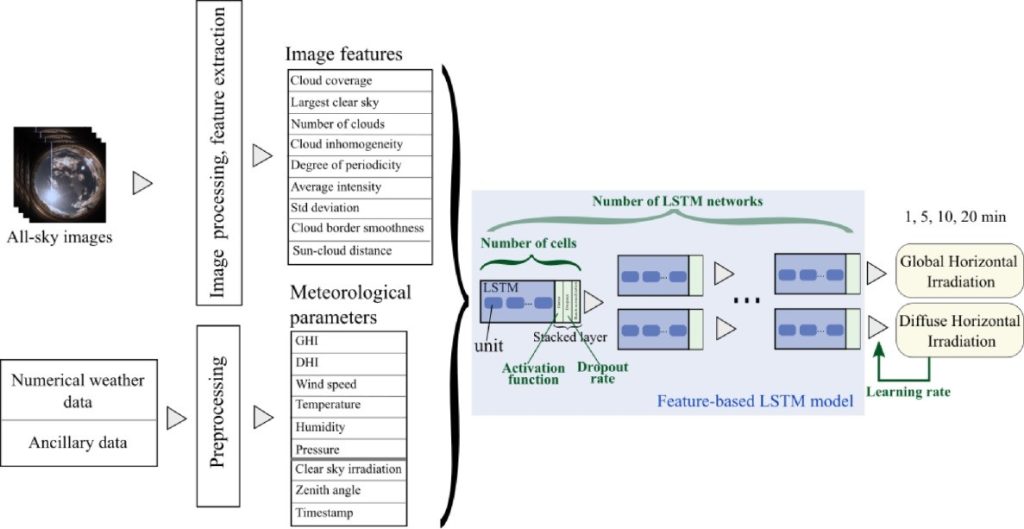
The model successfully predicted solar irradiance up to 20 minutes ahead, including rapid changes caused by moving clouds—so-called “ramp events” that are notoriously hard to predict. This kind of ultra-short-term forecast is crucial for improving the operation of solar panels, battery storage systems, and power grids, especially as renewable energy continues to grow.
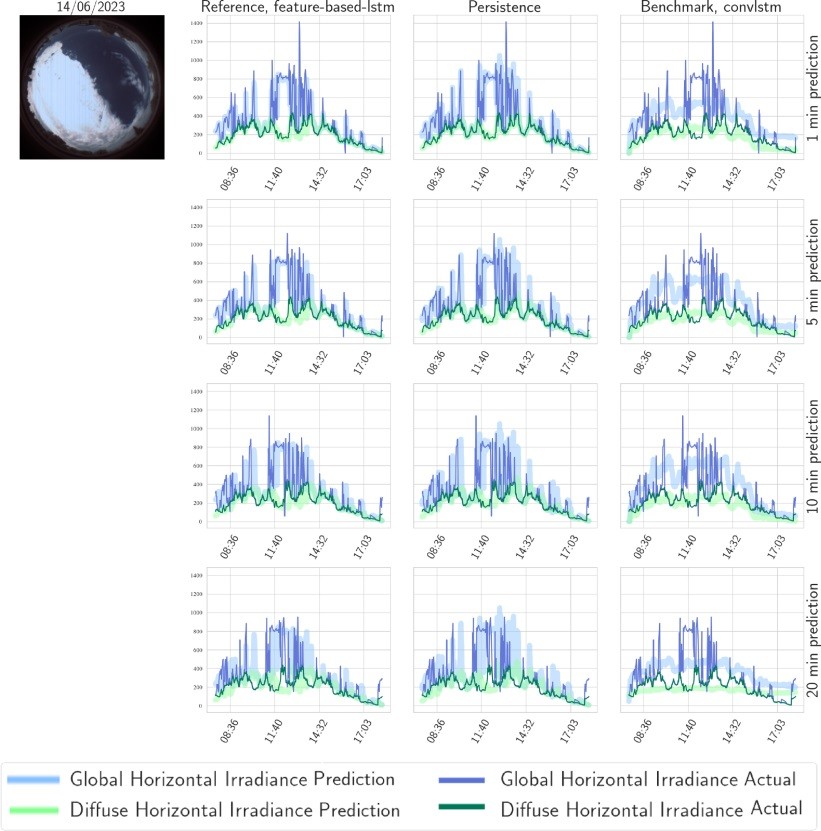
Thanks to its simplicity and speed, the method is ideal for use in remote sensors or edge devices where computing power is limited, and it could help energy system operators better manage solar input and boost the reliability of green power grids.
This innovative model brings us one step closer to a smarter, cleaner energy future.
Barancsuk, L., Groma, V., & Kocziha, B. (2025). Hybrid ultra-short term solar irradiation forecasting using resource-efficient multi-step long-short term memory. Renewable Energy, 122962. (https://doi.org/10.1016/j.renene.2025.122962)